- AI and Machine Learning,
- Cybersecurity Training,
- Government Cybersecurity,
- SIEM (Security Information and Event Management)
AI Agents in SIEM: Transforming Fraud Detection and Cyber Defense
AI agents in SIEM enhance fraud detection and cyber defense by automating analytics, detecting anomalies, and reducing false positives. Using tools like Elastic ML, Ludwig, and MLFlow, Bellator Technologies LLC leverages AI-driven SIEM to strengthen security and stay ahead of threats.
Traditional Security Information and Event Management (SIEM) solutions rely on predefined rules and signature-based detection, which are effective but limited in handling dynamic, evolving fraud patterns. AI agents enhance SIEM capabilities by:
- Automating threat detection using anomaly detection models.
- Reducing false positives with contextual analysis.
- Adapting to new attack patterns through reinforcement learning.
- Enhancing threat hunting by analyzing behavioral patterns in real-time.
AI-Powered SIEM Use Case: Fraud Detection
Fraud detection requires analyzing vast amounts of data from financial transactions, user behavior, access logs, and system anomalies. AI agents can help in:
- Behavioral Analysis: AI tracks deviations in user behavior to detect suspicious activity (e.g., account takeovers, credential stuffing).
- Real-Time Anomaly Detection: Machine learning models identify unusual transactions based on historical data.
- Automated Incident Response: AI agents trigger alerts and initiate investigation workflows without human intervention.
- Pattern Recognition for Fraud Rings: AI clusters fraudulent behaviors to detect organized cybercrime operations.
How to Integrate AI Agents into Your SIEM
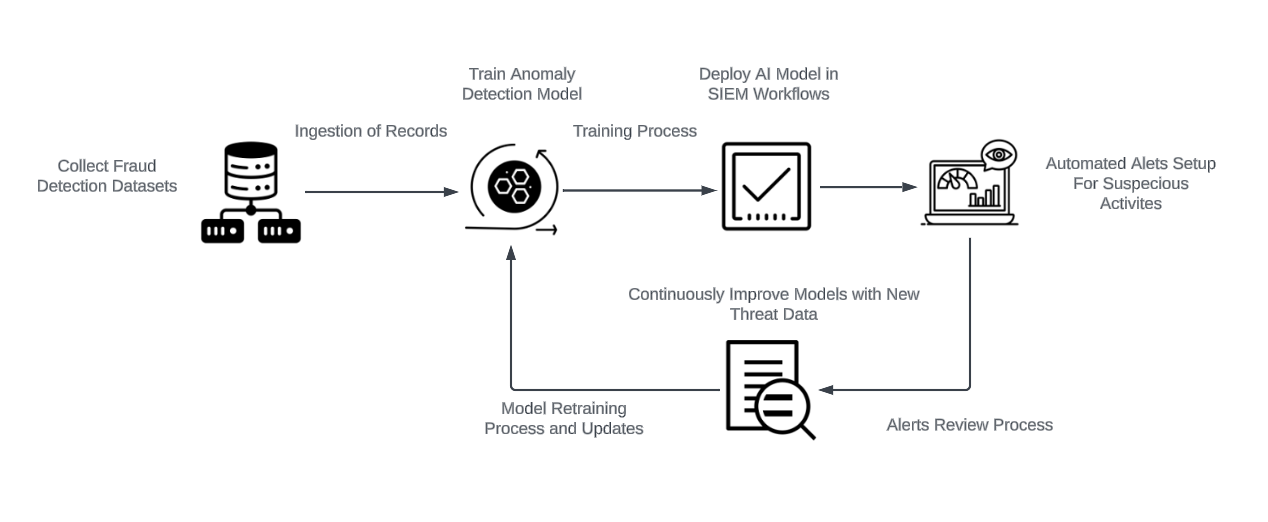
Step 1: Collect fraud detection datasets (e.g., from financial logs, authentication logs).
Step 2: Train an anomaly detection model using Elastic ML, Ludwig, or MLFlow.
Step 3: Deploy the AI model within SIEM workflows to classify events in real-time.
Step 4: Set up automated alerts for suspicious activities detected by AI.
Step 5: Continuously improve models by retraining with new threat data.
These steps form the fundamental framework for integrating AI-driven fraud detection into a SIEM system. While they appear straightforward, they require substantial data preparation, validation, and iterative improvements to be effective.
Letβs Build an AI-Powered SIEM Together
Whether you're looking to optimize an existing SIEM or explore AI-driven security analytics, our team can help. We provide custom AI integrations, tailored workflows, and expert-driven cybersecurity solutions that align with your organization's security and compliance needs. π
π© Connect with Us Today!
π Schedule a consultation to discuss how AI-powered SIEM can strengthen your security posture.
π§ Contact Bellator Technologies LLC to get started.
π Stay ahead of emerging threats with AI-driven cybersecurity innovation!